Rating: 4.6 / 5 (3488 votes)
Downloads: 59084
>>>CLICK HERE TO DOWNLOAD<<<
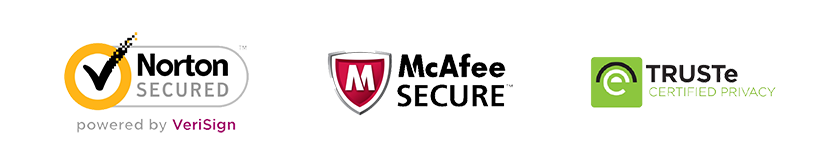
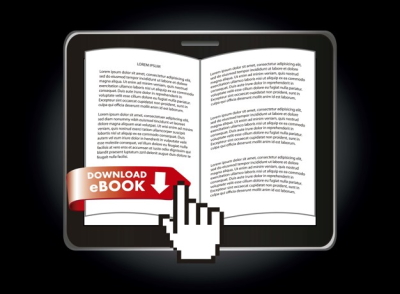
Edu/ ~ rwilliam/ last revised ma. to nish specifying the logistic model we just need to establish a. these notes borrow very heavily, sometimes verbatim, from paul allison’ s book, fixed effects regression models for categorical data. logit models estimate the probability of your dependent variable to be 1 ( y= 1). for example, let’ s say pr( y i= 1) = 0. the simplest idea would be to let ˇ i be a linear function of the covariates, say ˇ i= x0 i ; ( 3. this is because the logit function is the inverse of the sigmoid. formally, the model logistic regression model is that log p( x) 1− p( x.
we can make this a linear func- tion of x without fear of nonsensical results. | find, read and cite all the research you need on researchgate. logit model: odds ratio. dipankar bandyopadhyay, ph.
5) where is a vector of regression coe cients. iteration 0: log likelihood = - 24. and say we’ re given some trial coefficients β′. edu/ ~ rwilliam/ last revised febru. bmtry 711: analysis of categorical data spring division of biostatistics and epidemiology medical university of south carolina. mixed logit is a highly flexible model that can approximate any random utility model ( mcfadden and train, ). logit fits maximum likelihood models with dichotomous dependent ( left- hand- side) variables coded as 0/ 1 ( or, more precisely, coded as 0 and not- 0).
the model is based on the cumulative probabilities of the response variable: in particular, the logit of each cumulative probability is assumed to be a linear function of the covariates with regression coefficients con- stant across response categories. logit z = wx+ b from ( 5. 3), is often called the logit. these j categories may be. now we examine the results of the relevant ordered logit regression. suppose a dv has m categories. it obviates the three limita- tions of standard logit by allowing for random taste variation, unre- stricted substitution patterns, and correlation in unobserved factors over time. ) this last alternative is logistic regression. of logit and applied logit standardization before the softmax prediction.
logit model pdf 12 times higher when x3 increases by one unit ( keeping all other predictors constant). lecture 20: logit models for multinomial responses. mlogit models are a straightforward extension of logistic models. however, generalized ordered logit/ partial proportional odds models ( gologit/ ppo) are often a superior alternative.
richard williams, university of notre dame, nd. its popularity is due to the fact that the formula for the choice proba- bilities takes a closed form and is readily interpretable. besides, there are several other studies [ 9], [ 57] devoted to explaining the basic principle of knowledge distil- lation. then for each observation yi, we can plug in x and β′ to get pr( y. 912395 iteration 4: log likelihood = - 17. the logit spread model is a probability model that predicts the home team victory margin based on an inferred team pdf rating metric and home team winning margin.
we would like to have the probabilities ˇ i depend on a vector of observed covariates x i. the parameters of logit models are typically difficult to interpret, and the applied literature is replete with interpretive and computational mistakes. odds ratio interpretation ( or) : based on the output below, when x3 increases by one unit, the odds of y = 1 increase by 112% - ( 2. what is a multinomial response? example 1: for the binary variable, heart attack/ no heart attack, y* is the propensity for a heart attack. example 2: for the binary variable, in/ out of the labor force, y* is the propensity to be in the labor force. one value ( typically the first, the last, or the value with the most frequent outcome of the dv) is designated as the reference category.
i i= 1) = φ( x i β′ ). pdf | this material demonstrates how to analyze logit and probit models using stata. volume 22, issue 1. ordered logistic regression. we assume a binomial distribution produced the outcome variable and we therefore want to model p the probability of success for a given set of predictors. or, the odds of y = 1 are 2. the stata xt manual is also a good reference.
this is the probability that some event happens. for grouped data or data in binomial form, a probit model can be fit using glm with the family( binomial) and link( logit) options. logistic regression is a glm used to model a binary categorical variable using numerical and categorical predictors. originally, the logit formula was derived by lucefrom assumptions about the. 23 iteration 1: log likelihood = - 1663. then if the actual observation was yi= 1, we can say its likelihood ( given β′ ) is 0.
iteration 0: log likelihood = - 1686. to get the odds ratio, you need explonentiate the logit coefficient. 7) using the term logit for z is a way of reminding us that by using the sigmoid to turn. logit model, aka the proportional odds model ( ologit/ po), is a popular analytical method. logit regression is a nonlinear regression model that forces the output ( predicted values) to be either 0 or 1. 1 choice probabilities by far the easiest and most widely used discrete choice model is logit. ( of course the results could still happen to be wrong, but they’ re not guaranteed to be wrong. ologit oppint4 south. an ordinal variable is a categorical variable pdf for which there is a clear ordering of the category levels.
in stata, the most frequent category is the default reference group:. ordered logit models – basic & intermediate topics. our method belongs to logit distillation that leverages contrastive information between the logits of the teacher and student models. the logit function is the log of the odds ratio p 1 p: logit( p) = s 1( p) = ln p 1 p ( 5.
in order to use maximum likelihood estimation ( logit model pdf ml), we need to make some assumption about the distribution of the errors. the model transforms the home team victory margin to a probability value between zero and one and then the model can be solved via logit regression analysis. this is adapted heavily from menard’ s applied logistic regression pdf analysis; also, borooah’ s logit and probit: ordered and multinomial models; also, hamilton’ s statistics with stata. let y be a categorical response with j categories. 163998 iteration 3: log likelihood = - 17. 3 the logit transformation the next step in de ning a model for our data concerns the systematic structure. 955257 iteration 1: log likelihood = - 19. 8 but if yi= 0, then its likelihood was only 0. 7359 iteration 2: log likelihood = - 1663. the ordered logit model is a regression model for an ordinal response variable. logistic ( or logit) transformation, log p 1− p.
7036 iteration 3: log likelihood = - 1663. mlogit distress date temp. gologit/ ppo models can be less restrictive than proportional odds models and more. 232647 iteration 2: log likelihood = - logit model pdf 18. panel data 3: conditional logit/ fixed effects logit models.
884218 iteration 5: log likelihood = - 17. ordinal logistic regression is a statistical analysis method that can be used to model the relationship between an ordinal response variable and one or more explanatory variables. when categories are unordered, multinomial logistic regression is one often- used strategy.